[ad_1]
The essential to building versatile device-finding out products that are able of reasoning like men and women do may not be feeding them oodles of education details. As a substitute, a new review implies, it could appear down to how they are qualified. These findings could be a large move toward better, much less mistake-prone synthetic intelligence types and could assistance illuminate the secrets and techniques of how AI systems—and humans—learn.
People are master remixers. When folks realize the relationships among a established of factors, this sort of as foods elements, we can mix them into all kinds of mouth watering recipes. With language, we can decipher sentences we have in no way encountered right before and compose advanced, authentic responses for the reason that we grasp the underlying meanings of terms and the rules of grammar. In technological terms, these two illustrations are proof of “compositionality,” or “systematic generalization”—often viewed as a important theory of human cognition. “I believe that is the most crucial definition of intelligence,” states Paul Smolensky, a cognitive scientist at Johns Hopkins University. “You can go from being aware of about the parts to dealing with the whole.”
Accurate compositionality may possibly be central to the human head, but machine-learning builders have struggled for decades to show that AI methods can reach it. A 35-calendar year-previous argument manufactured by the late philosophers and cognitive experts Jerry Fodor and Zenon Pylyshyn posits that the principle may well be out of attain for typical neural networks. Today’s generative AI models can mimic compositionality, manufacturing humanlike responses to published prompts. However even the most state-of-the-art versions, which includes OpenAI’s GPT-3 and GPT-4, nonetheless tumble limited of some benchmarks of this skill. For occasion, if you check with ChatGPT a concern, it may possibly at first provide the suitable remedy. If you proceed to ship it comply with-up queries, on the other hand, it could possibly fall short to stay on topic or start out contradicting alone. This suggests that though the types can regurgitate information from their schooling information, they never definitely grasp the indicating and intention powering the sentences they produce.
But a novel education protocol that is focused on shaping how neural networks discover can increase an AI model’s capacity to interpret data the way human beings do, according to a examine published on Wednesday in Mother nature. The results advise that a sure approach to AI schooling may well build compositional equipment learning versions that can generalize just as properly as people—at least in some occasions.
“This research breaks essential ground,” claims Smolensky, who was not concerned in the study. “It accomplishes a little something that we have required to attain and have not beforehand succeeded in.”
To coach a program that appears to be able of recombining parts and comprehension the indicating of novel, elaborate expressions, scientists did not have to make an AI from scratch. “We did not need to have to essentially adjust the architecture,” says Brenden Lake, direct writer of the research and a computational cognitive scientist at New York University. “We just experienced to give it observe.” The scientists commenced with a standard transformer model—a model that was the very same sort of AI scaffolding that supports ChatGPT and Google’s Bard but lacked any prior textual content instruction. They ran that essential neural community by means of a specially made established of tasks meant to instruct the system how to interpret a manufactured-up language.
The language consisted of nonsense text (this kind of as “dax,” “lug,” “kiki,” “fep” and “blicket”) that “translated” into sets of vibrant dots. Some of these invented text were symbolic conditions that specifically represented dots of a sure shade, while some others signified functions that improved the purchase or quantity of dot outputs. For instance, dax represented a uncomplicated crimson dot, but fep was a operate that, when paired with dax or any other symbolic phrase, multiplied its corresponding dot output by a few. So “dax fep” would translate into 3 purple dots. The AI instruction provided none of that data, however: the scientists just fed the model a handful of examples of nonsense sentences paired with the corresponding sets of dots.
From there, the examine authors prompted the design to develop its possess collection of dots in response to new phrases, and they graded the AI on whether or not it experienced appropriately adopted the language’s implied principles. Soon the neural community was capable to react coherently, next the logic of the nonsense language, even when released to new configurations of phrases. This implies it could “understand” the made-up procedures of the language and utilize them to phrases it hadn’t been skilled on.
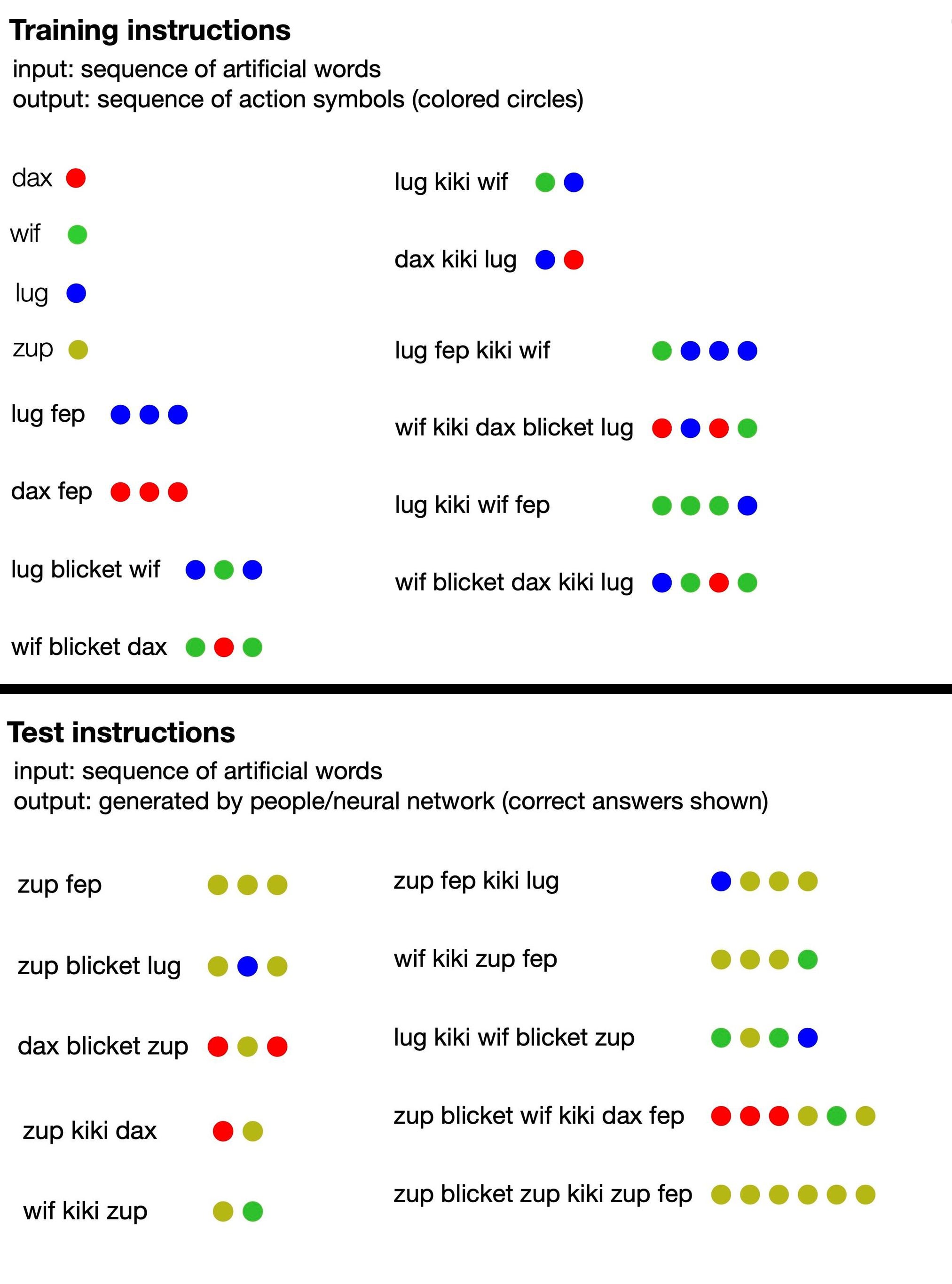
On top of that, the researchers analyzed their skilled AI model’s knowledge of the designed-up language towards 25 human members. They discovered that, at its finest, their optimized neural community responded 100 p.c accurately, although human solutions were being proper about 81 percent of the time. (When the workforce fed GPT-4 the teaching prompts for the language and then requested it the check issues, the significant language model was only 58 per cent precise.) Provided added education, the researchers’ conventional transformer product begun to mimic human reasoning so perfectly that it made the same errors: For instance, human members usually erred by assuming there was a a person-to-one particular partnership among specific phrases and dots, even though numerous of the phrases didn’t observe that sample. When the product was fed illustrations of this conduct, it immediately started to replicate it and built the mistake with the exact frequency as people did.
The model’s efficiency is significantly extraordinary, provided its tiny dimension. “This is not a big language product educated on the total Online this is a somewhat small transformer experienced for these tasks,” suggests Armando Solar-Lezama, a laptop scientist at the Massachusetts Institute of Technology, who was not associated in the new review. “It was attention-grabbing to see that nevertheless it is able to show these kinds of generalizations.” The locating implies that as a substitute of just shoving ever more education knowledge into device-understanding models, a complementary approach may be to supply AI algorithms the equal of a concentrated linguistics or algebra course.
Solar-Lezama states this instruction process could theoretically give an alternate route to greater AI. “Once you’ve fed a model the full World-wide-web, there is no next World-wide-web to feed it to further strengthen. So I think procedures that pressure styles to explanation superior, even in artificial jobs, could have an impact heading ahead,” he says—with the caveat that there could be issues to scaling up the new coaching protocol. At the same time, Photo voltaic-Lezama thinks these scientific tests of smaller sized models assistance us better realize the “black box” of neural networks and could lose mild on the so-referred to as emergent capabilities of much larger AI techniques.
Smolensky provides that this research, alongside with very similar operate in the long term, might also improve humans’ understanding of our very own brain. That could enable us style and design systems that decrease our species’ error-prone tendencies.
In the existing, however, these positive aspects stay hypothetical—and there are a pair of large limitations. “Despite its successes, their algorithm doesn’t remedy every obstacle lifted,” states Ruslan Salakhutdinov, a laptop or computer scientist at Carnegie Mellon College, who was not included in the analyze. “It does not instantly deal with unpracticed kinds of generalization.” In other phrases, the schooling protocol assisted the design excel in a single form of undertaking: studying the patterns in a faux language. But presented a entire new undertaking, it could not apply the same skill. This was obvious in benchmark exams, where by the design unsuccessful to control for a longer period sequences and could not grasp earlier unintroduced “words.”
And crucially, each individual qualified Scientific American spoke with noted that a neural network capable of constrained generalization is quite different from the holy grail of artificial typical intelligence, whereby computer versions surpass human capability in most duties. You could argue that “it’s a really, very, pretty little stage in that way,” Photo voltaic-Lezama suggests. “But we’re not speaking about an AI buying capabilities by alone.”
From restricted interactions with AI chatbots, which can present an illusion of hypercompetency, and considerable circulating buzz, lots of people today may perhaps have inflated suggestions of neural networks’ powers. “Some men and women might obtain it surprising that these forms of linguistic generalization duties are actually really hard for systems like GPT-4 to do out of the box,” Solar-Lezama says. The new study’s results, nevertheless interesting, could inadvertently serve as a reality check out. “It’s seriously important to continue to keep observe of what these devices are able of doing,” he says, “but also of what they simply cannot.”
[ad_2]
Source url